Hi! Today I would like to tell you what kind of people make up our sports analytics team at CNR Analytics.
And I will briefly tell you what algorithms we use to predict accurate match outcomes!
We have a team of professionals working in the background who take sports analytics up a notch at CNR Analytics. We will give you an introduction to the people behind the company sports analytics team and provide insight into their operations. These analysts cover it all — from soccer showdowns to cricket clashes, from the boxing ring to the hockey rink. Each member of the team has a remote job in different time zone, however they manage to work closely in predicting the game results for betting fans from all around the globe.
Let's get acquainted, my name is Mike Clay and I am the editor-in-chief and website and social media analyst for CNR Analytics. For over 20 years I have been in this line of work. All significant decisions go through me.
And I will briefly tell you what algorithms we use to predict accurate match outcomes!
We have a team of professionals working in the background who take sports analytics up a notch at CNR Analytics. We will give you an introduction to the people behind the company sports analytics team and provide insight into their operations. These analysts cover it all — from soccer showdowns to cricket clashes, from the boxing ring to the hockey rink. Each member of the team has a remote job in different time zone, however they manage to work closely in predicting the game results for betting fans from all around the globe.
Let's get acquainted, my name is Mike Clay and I am the editor-in-chief and website and social media analyst for CNR Analytics. For over 20 years I have been in this line of work. All significant decisions go through me.
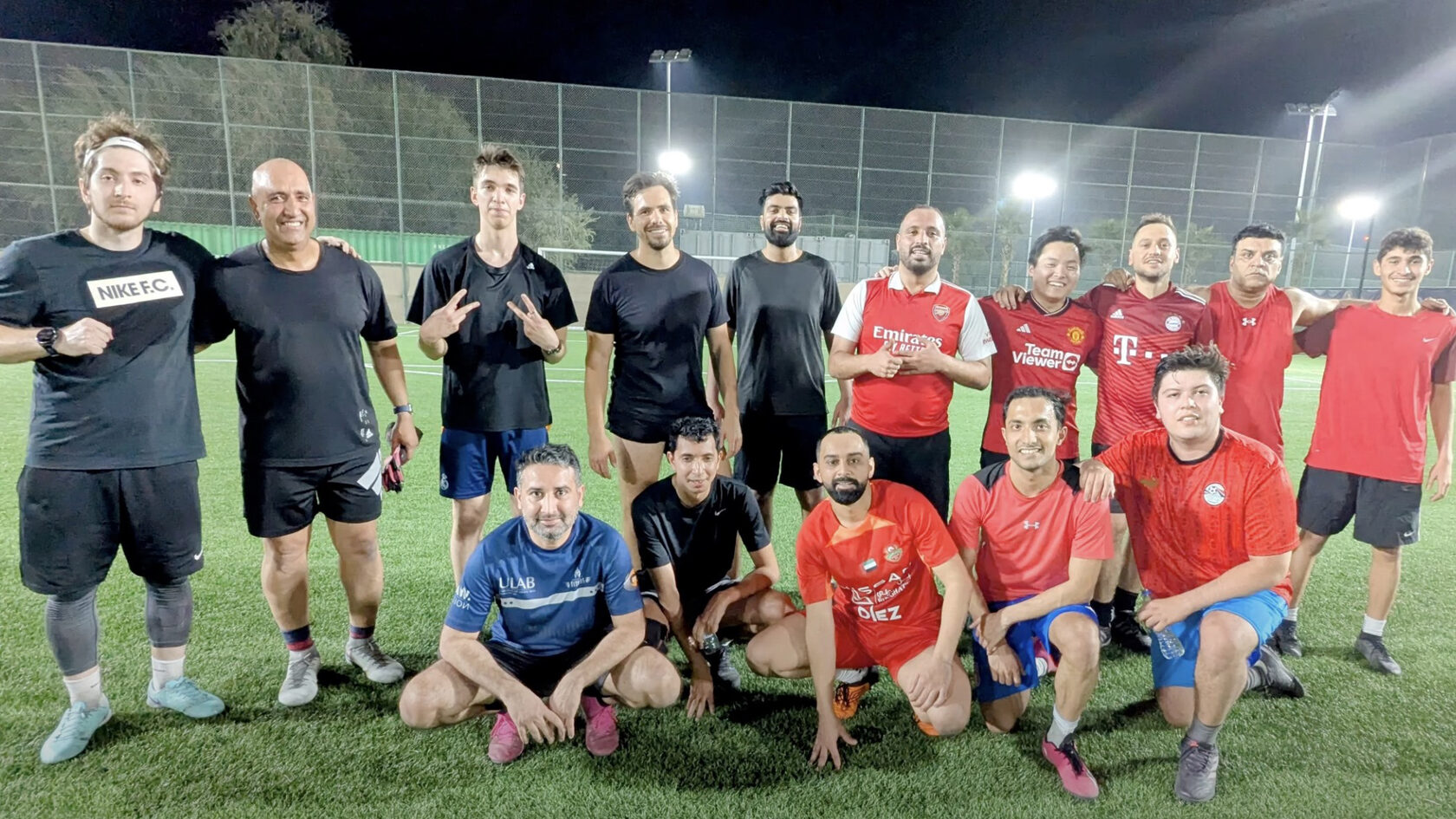
About the company
Official name is Analytical Solutions and the company is headquartered in New Zealand, Wellington. But many of our employees work remotely in different cities around the world.
Most of the team involved in management, design and marketing live in New Zealand. We also often organize amateur football matches after work.
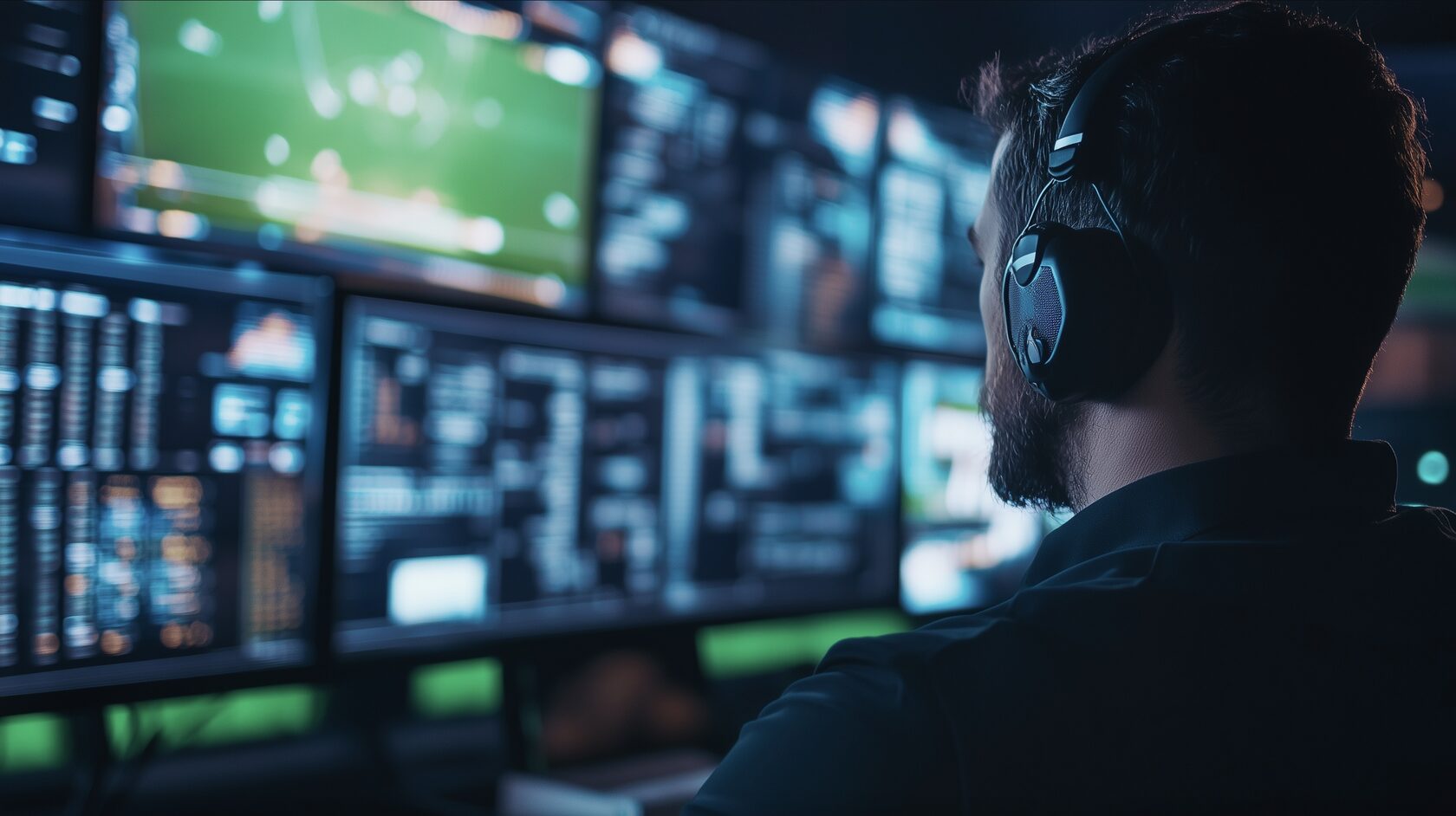
Football Analytics Team
Liam O'Connor (New Zealand, Wellington) is a senior soccer analyst specializing in European league predictions (Premier League and UEFA competitions). Liam uses Python and advanced statistical models to predict match outcomes for betting purposes, working remotely from London and ensuring each prediction is grounded in data.
Camila Neves (São Paulo, Brazil) — Data scientist focusing on South-American football analytics and player performance statistics. Camila works remotely out of São Paulo and usses a machine learning algorithm and local league data sets to objectively predict behavour of betting markets – so all her match predictions are data driven and as professional as possible.
Diego Marquez (Buenos Aires, Argentina) is a football stats expert focused on pricing models to calculate a players' goal score and World Cup historical data. Diego applies statistics-heavy predictive models that predict the outcome of games for betting purposes, and infuses Latin American insights while working from Buenos Aires with each prediction backed by rigorous stats.
Amina Farouk (Cairo, Egypt) is a sports data analyst specializing in African and Middle Eastern leagues. Amina relies on rich data visualization and Python scripting to generate accurate predictions for betting markets. She operates remotely from Cairo, bringing data integrity and a diverse league perspective to every soccer forecast.
Camila Neves (São Paulo, Brazil) — Data scientist focusing on South-American football analytics and player performance statistics. Camila works remotely out of São Paulo and usses a machine learning algorithm and local league data sets to objectively predict behavour of betting markets – so all her match predictions are data driven and as professional as possible.
Diego Marquez (Buenos Aires, Argentina) is a football stats expert focused on pricing models to calculate a players' goal score and World Cup historical data. Diego applies statistics-heavy predictive models that predict the outcome of games for betting purposes, and infuses Latin American insights while working from Buenos Aires with each prediction backed by rigorous stats.
Amina Farouk (Cairo, Egypt) is a sports data analyst specializing in African and Middle Eastern leagues. Amina relies on rich data visualization and Python scripting to generate accurate predictions for betting markets. She operates remotely from Cairo, bringing data integrity and a diverse league perspective to every soccer forecast.
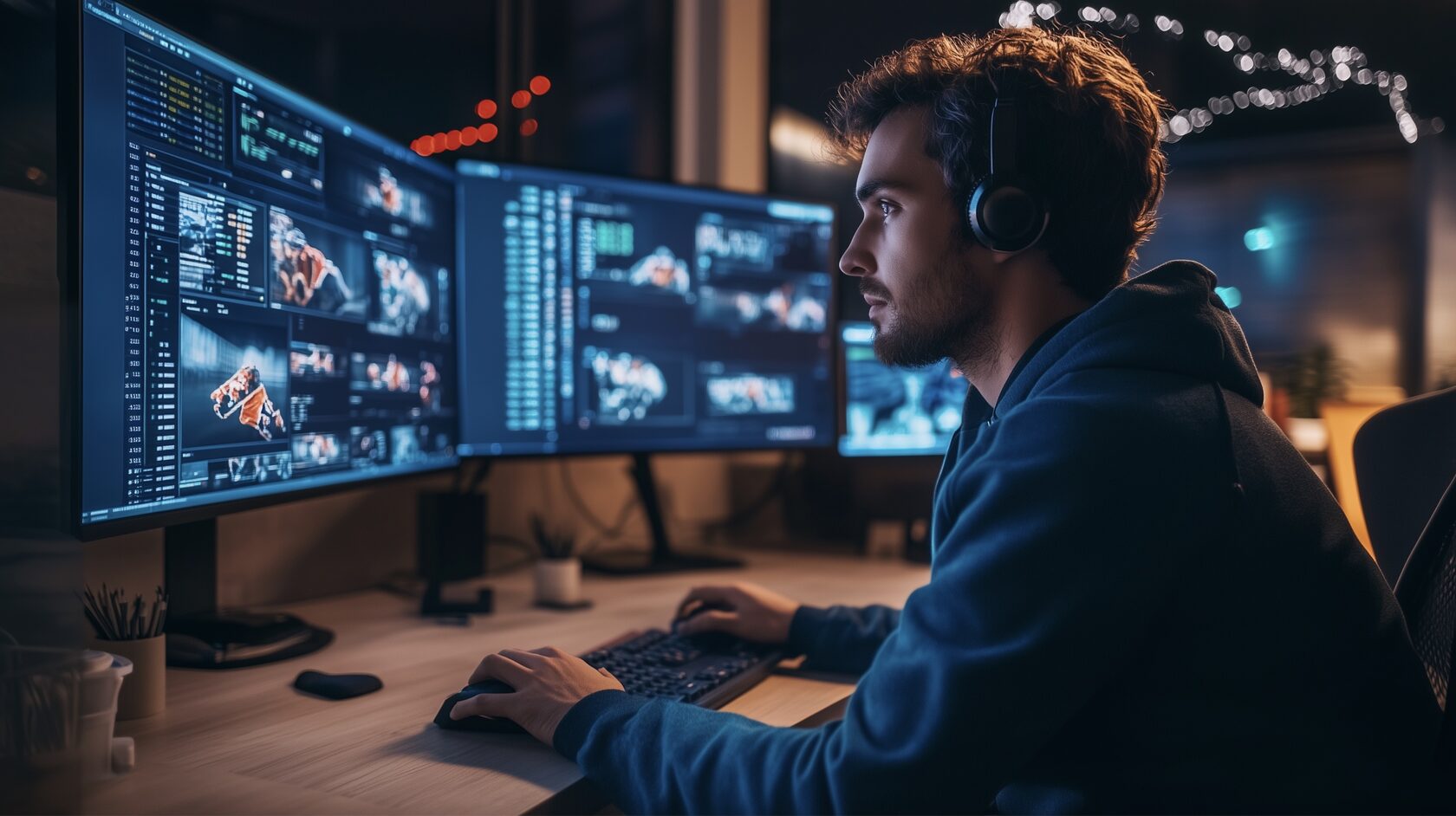
UFC—Boxing Analytics Team
Travis King (Las Vegas, USA) — A wrestling and sports scientist background, Travis leads the UFC-Boxing analytics efforts. Travis is an expert in mixed martial arts data modeling, with a focus on how different fighting styles have played out in past fights. He predicts fight outcomes and spots value in betting odds for UFC events using Python and machine-learning frameworks while working remotely out of Las Vegas.
Bruno Almeida (Rio de Janeiro, Brazil) — A combat sports data analyst with emphasis on the impact of MMA and Brazilian Jiu-Jitsu on fight results. Using a broad dataset of UFC bouts, Bruno taps into trends such as takedown success rates and striking accuracy. He lives from home in Rio, using R and Python (via pandas and scikit-learn) to generate wagering forecasts with a Brazilian eye on the sport.
Irina Petrova is an MMA-Boxing analytics expert who brings a rigorous statistical approach to fight analysis. Irina specializes in evaluating fighters' performance trends and stamina over time. Operating remotely from Moscow, she employs predictive models and even neural networks to forecast UFC match results for betting purposes. Her work blends international MMA insights with cutting-edge data science techniques.
Bruno Almeida (Rio de Janeiro, Brazil) — A combat sports data analyst with emphasis on the impact of MMA and Brazilian Jiu-Jitsu on fight results. Using a broad dataset of UFC bouts, Bruno taps into trends such as takedown success rates and striking accuracy. He lives from home in Rio, using R and Python (via pandas and scikit-learn) to generate wagering forecasts with a Brazilian eye on the sport.
Irina Petrova is an MMA-Boxing analytics expert who brings a rigorous statistical approach to fight analysis. Irina specializes in evaluating fighters' performance trends and stamina over time. Operating remotely from Moscow, she employs predictive models and even neural networks to forecast UFC match results for betting purposes. Her work blends international MMA insights with cutting-edge data science techniques.
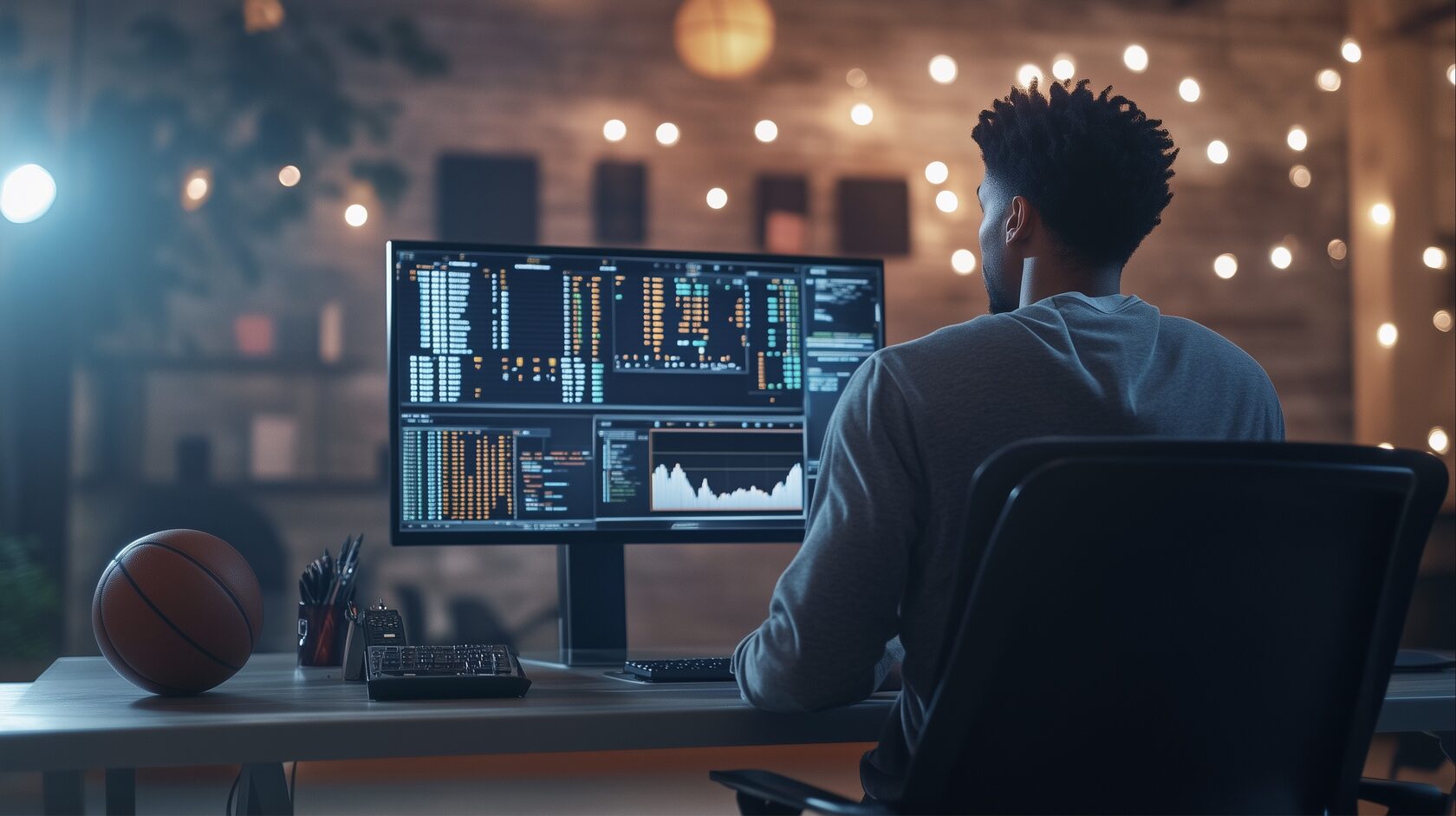
Basketball Analytics Team
Jordan Thompson (Chicago, USA) is the head basketball analyst specializing in the NBA, along with advanced metrics such as player efficiency ratings and win shares. Jordan is working remotely in Chicago, analyzing large NBA datasets with Python and SQL. His specialty is predictive modeling for game results and point spreads to give gamblers data-driven forecasts for basketball games.
Emily Brown (Toronto, Canada) — statistician and bball data expert covering NBA and international. Emily is from Toronto, working remotely, using a lot of R for statistical analysis and visualization of trends in team performance. Her specialty is finding obscure elements (travel fatigue or back-to-back game effects, for instance) that may alter the outcome of a game and then using those discoveries to make intelligent wagering predictions.
Nikhil Sharma (Mumbai, India) — NBA analytics geek turned professional data analyst at CNR Analytics. Based out of Mumbai while working remotely, Nikhil concentrates on connecting the dots for Asian popcorns. And his predictions for game outcomes use machine learning models in Python (including TensorFlow for complex pattern recognition). His analyses often show how small statistical differences, like pace-of-play or turnover ratios, can alter betting odds.
Sofia Martinez (Madrid, Spain): a basketball analytics guru who will be watching the European leagues and international tournaments closely. Sofia — who works remotely from Madrid — relies on pandas or scikit-learn for game data analysis, with most of her work focused on teams that participate in EuroLeague or FIBA competitions. She brings a global perspective to CNR Analytics basketball predictions, administering a thorough data analysis process before you place your next NBA or global hoop bet.
Emily Brown (Toronto, Canada) — statistician and bball data expert covering NBA and international. Emily is from Toronto, working remotely, using a lot of R for statistical analysis and visualization of trends in team performance. Her specialty is finding obscure elements (travel fatigue or back-to-back game effects, for instance) that may alter the outcome of a game and then using those discoveries to make intelligent wagering predictions.
Nikhil Sharma (Mumbai, India) — NBA analytics geek turned professional data analyst at CNR Analytics. Based out of Mumbai while working remotely, Nikhil concentrates on connecting the dots for Asian popcorns. And his predictions for game outcomes use machine learning models in Python (including TensorFlow for complex pattern recognition). His analyses often show how small statistical differences, like pace-of-play or turnover ratios, can alter betting odds.
Sofia Martinez (Madrid, Spain): a basketball analytics guru who will be watching the European leagues and international tournaments closely. Sofia — who works remotely from Madrid — relies on pandas or scikit-learn for game data analysis, with most of her work focused on teams that participate in EuroLeague or FIBA competitions. She brings a global perspective to CNR Analytics basketball predictions, administering a thorough data analysis process before you place your next NBA or global hoop bet.
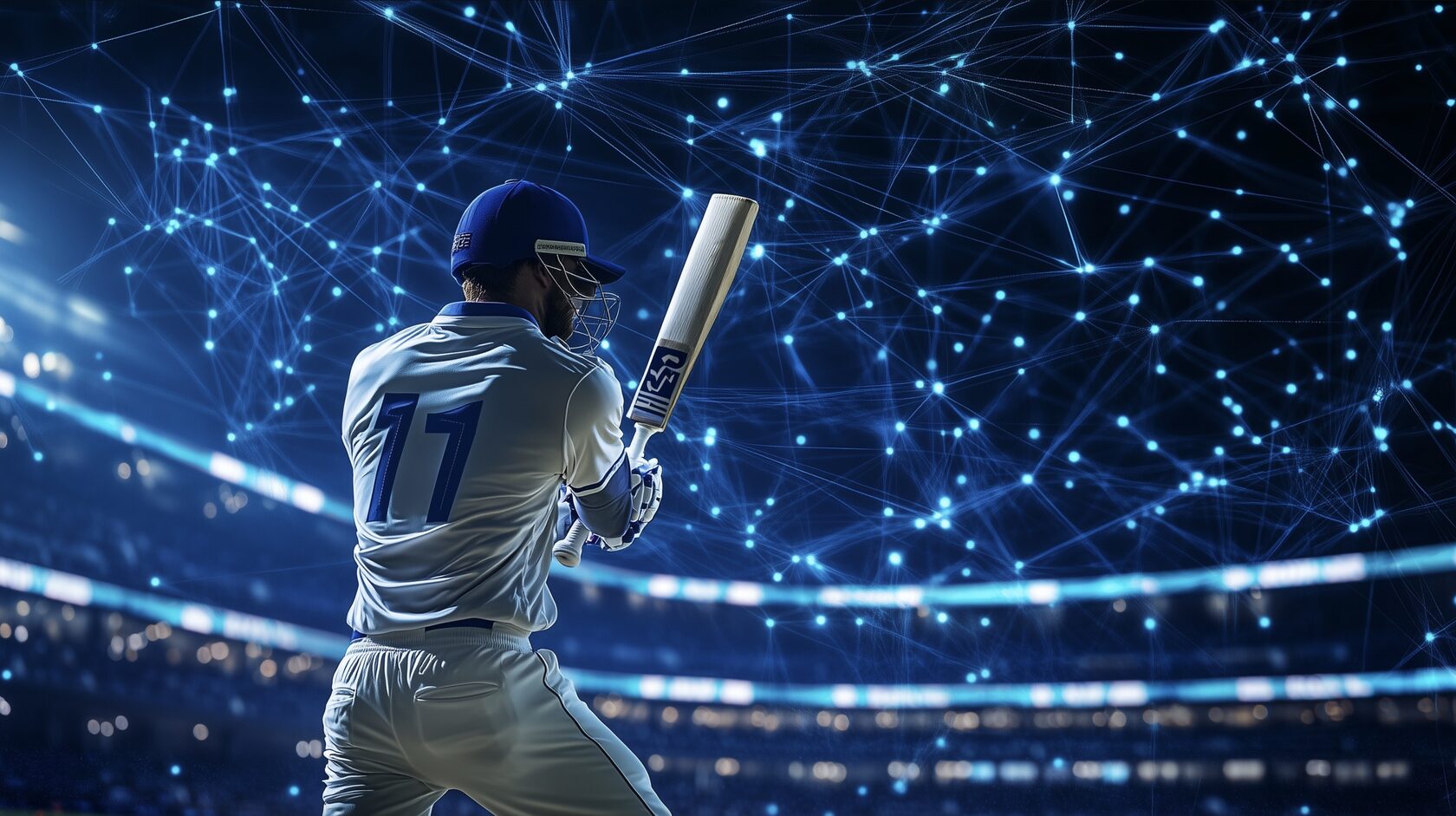
Cricket Analytics Team
Vikram Sharma (Mumbai, India) is a senior cricket analyst with a wealth of knowledge of statistics from Test matches to T20 leagues. Vikram works remotely from Mumbai and deals with large cricket datasets using Python and libraries like pandas and NumPy. He focuses on predictive models of match outcomes, and player performance in leagues like the IPL, applying techniques such as regression analysis and machine learning classifiers to help bettors gain an edge.
Rachel McCarthy (Sydney, Australia) is a sports data scientist who leads analysis of cricket in the Southern Hemisphere. Rachel works remotely from Sydney, specialising in Big Bash League and international cricket data. She uses R (specifically packages such as dplyr and caret) and cloud-computing resources to simulate match scenarios. Her technical skill with predictive analytics aids not only in forecasting outcomes but also in identifying probabilities for winning markets and betting.
James Walker (London, UK) is a cricket analytics specialist covering England and other commonwealth cricketing nations. James is a remote worker based in London who applies a more traditional sabermetric regime (think Moneyball, but for cricket) to the team. He fits predictive models in Python and R, examining not just pitch conditions and weather but also player form. Data-driven insights help him make clear odds predictions and betting tips for matches spanning from county games to World Cup finals.
Rachel McCarthy (Sydney, Australia) is a sports data scientist who leads analysis of cricket in the Southern Hemisphere. Rachel works remotely from Sydney, specialising in Big Bash League and international cricket data. She uses R (specifically packages such as dplyr and caret) and cloud-computing resources to simulate match scenarios. Her technical skill with predictive analytics aids not only in forecasting outcomes but also in identifying probabilities for winning markets and betting.
James Walker (London, UK) is a cricket analytics specialist covering England and other commonwealth cricketing nations. James is a remote worker based in London who applies a more traditional sabermetric regime (think Moneyball, but for cricket) to the team. He fits predictive models in Python and R, examining not just pitch conditions and weather but also player form. Data-driven insights help him make clear odds predictions and betting tips for matches spanning from county games to World Cup finals.
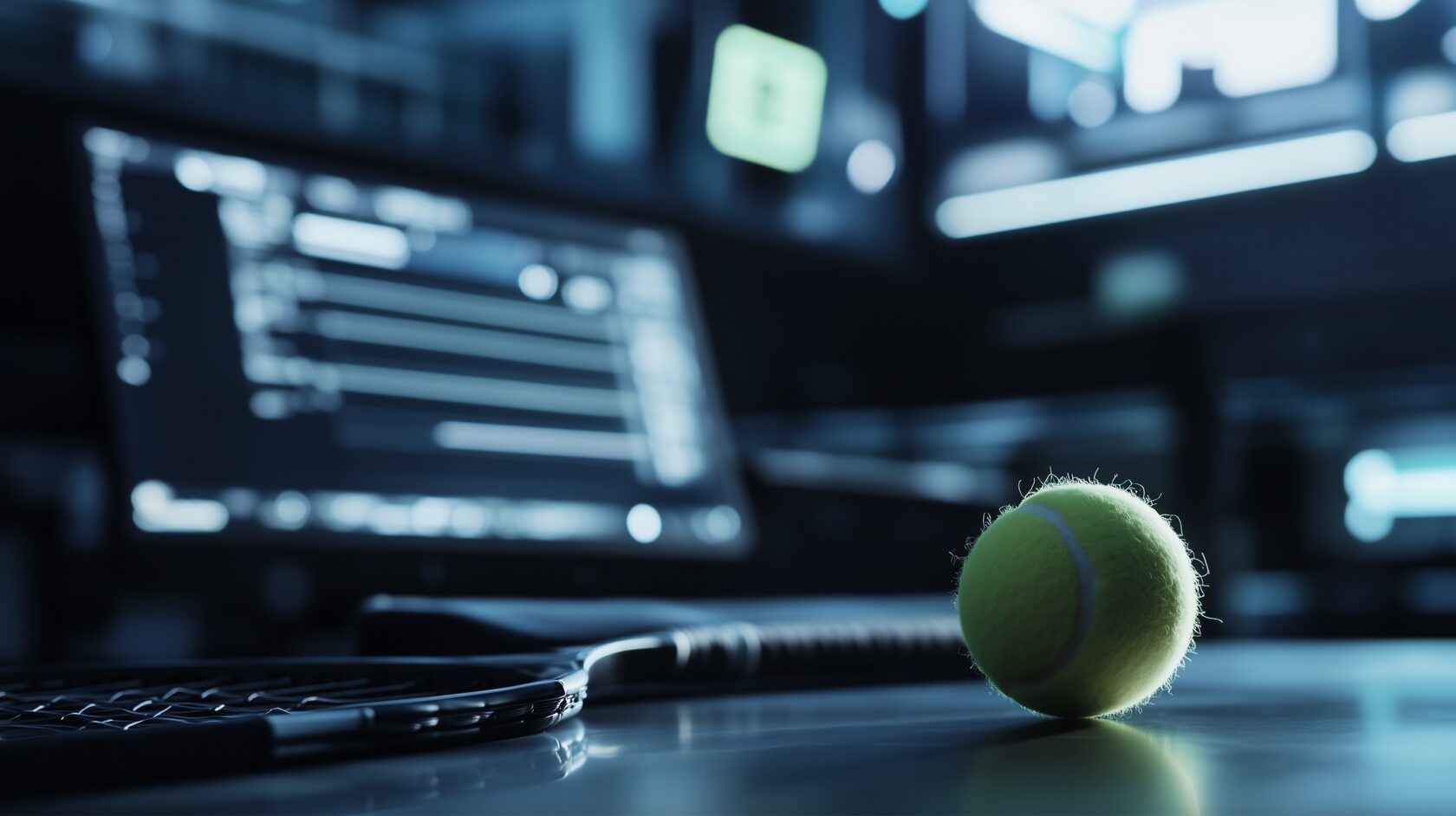
Tennis Analytics Team
Emily Carter (New York, USA) is a tennis analyst focusing on trends within Grand Slam tournaments and player performance. Based in New York and working remotely, Emily employs Python and statistical learning methods to examine serve velocities, rally lengths, and victory probabilities. Her work involves predictive modeling for match outcomes, using her data to help bettors understand what is likely to happen in high-stakes matches.
Alejandro Ruiz (Mexico City, Mexico) is a sports data analyst who introduces tennis analytics to the Spanish-speaking world. Alejandro works from home, from Mexico City, immersing himself in ATP and WTA tour data. He uses R to perform statistical analysis and Shiny dashboards to visualize player head-to-head comparisons and surface preferences. His expertise contributes predicting match outcomes and identifying betting value in tournaments from the Australian Open to the US Open, all with clear commentary that can be consumed around the world.
Priya Desai (Pune, India) is a tennis statistician who melds technical expertise with a love of the game. Priya works remotely from Pune and employs regression-based machine learning models (random forests and logistic regression in Python) to forecast match victors and even set-by-set probabilities. She focuses on how to integrate real-time data—like live match statistics—into the analysis pipeline. Her work aids timeline plays and offers detailed metrics in easily digestible forms.
Alejandro Ruiz (Mexico City, Mexico) is a sports data analyst who introduces tennis analytics to the Spanish-speaking world. Alejandro works from home, from Mexico City, immersing himself in ATP and WTA tour data. He uses R to perform statistical analysis and Shiny dashboards to visualize player head-to-head comparisons and surface preferences. His expertise contributes predicting match outcomes and identifying betting value in tournaments from the Australian Open to the US Open, all with clear commentary that can be consumed around the world.
Priya Desai (Pune, India) is a tennis statistician who melds technical expertise with a love of the game. Priya works remotely from Pune and employs regression-based machine learning models (random forests and logistic regression in Python) to forecast match victors and even set-by-set probabilities. She focuses on how to integrate real-time data—like live match statistics—into the analysis pipeline. Her work aids timeline plays and offers detailed metrics in easily digestible forms.
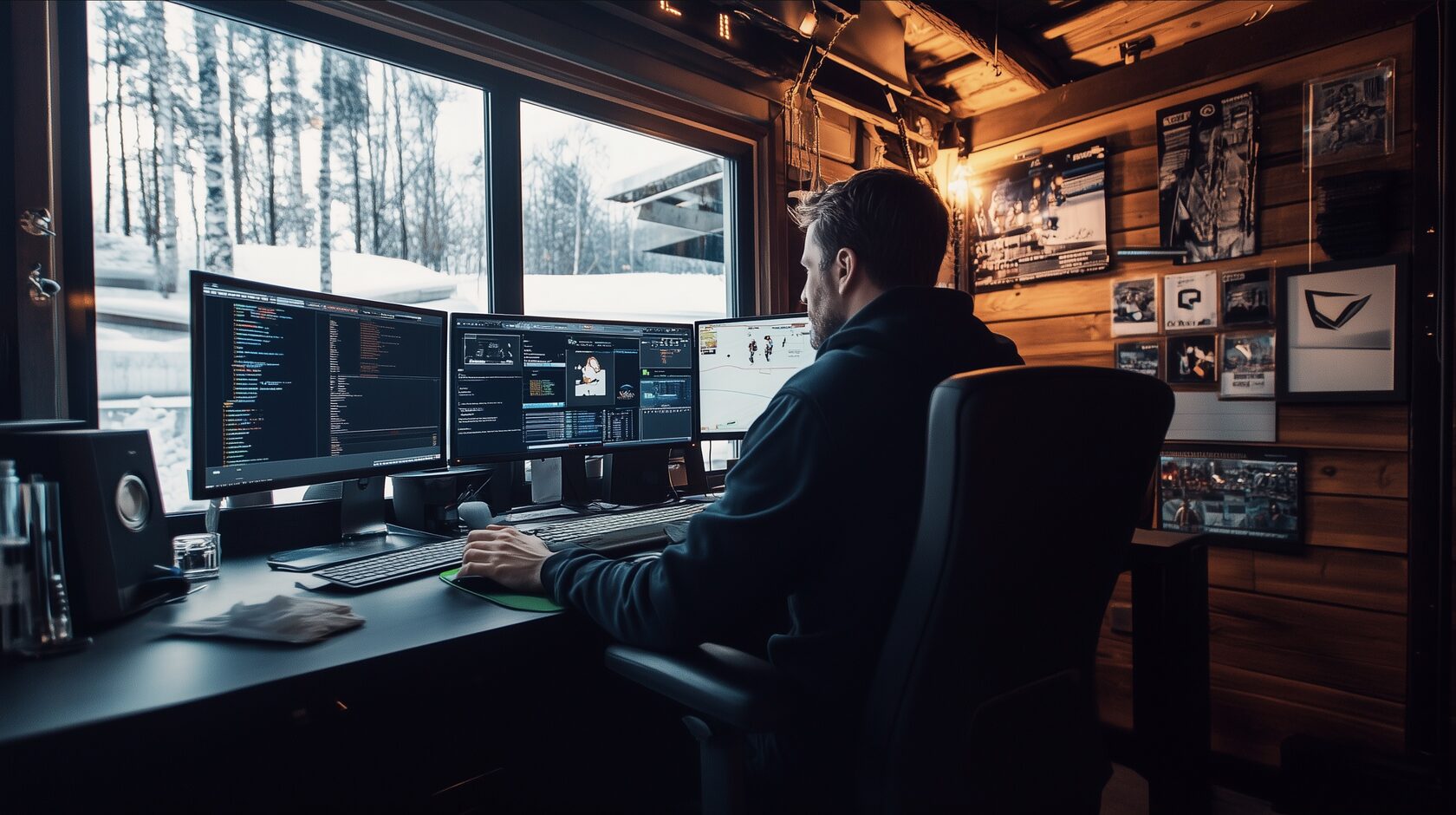
Hockey Analytics Team
Ethan Hughes (Boston, USA) Lead hockey analyst – specializing in NHL / player performance analytics Ethan has a background in statistics and works remotely from Boston. He employs Python and machine learning (including models for win probability and player impact) to explore how teams interact on the ice. His data-driven method for predicting game outcomes keeps bettors in line with hockey matchups.
Daniel Chen (Vancouver, Canada) is a data analyst and hockey fan who writes about North American and international ice hockey leagues. Based out of Vancouver, Daniel uses R, and big data tools to analyze past game data like shot on goal patterns and goalie performance analytics. He focuses on predicting game results and totals (over/under goals) for gambling, combining old-school hockey savvy with new-school math.
Alexei Morozov covers the NHL and the European leagues, such as the KHL — and brings a global perspective to the hockey analytics team. Alexei works out of Moscow, remotely, scraping data in python, and analyzing player stats in other continents. He uses predictive models to measure team momentum and fatigue factors. His model predicts what you can expect from both individual games and league championships, giving bettors a bird’s eye view that takes in multiple strengths of hockey traditions.
Daniel Chen (Vancouver, Canada) is a data analyst and hockey fan who writes about North American and international ice hockey leagues. Based out of Vancouver, Daniel uses R, and big data tools to analyze past game data like shot on goal patterns and goalie performance analytics. He focuses on predicting game results and totals (over/under goals) for gambling, combining old-school hockey savvy with new-school math.
Alexei Morozov covers the NHL and the European leagues, such as the KHL — and brings a global perspective to the hockey analytics team. Alexei works out of Moscow, remotely, scraping data in python, and analyzing player stats in other continents. He uses predictive models to measure team momentum and fatigue factors. His model predicts what you can expect from both individual games and league championships, giving bettors a bird’s eye view that takes in multiple strengths of hockey traditions.
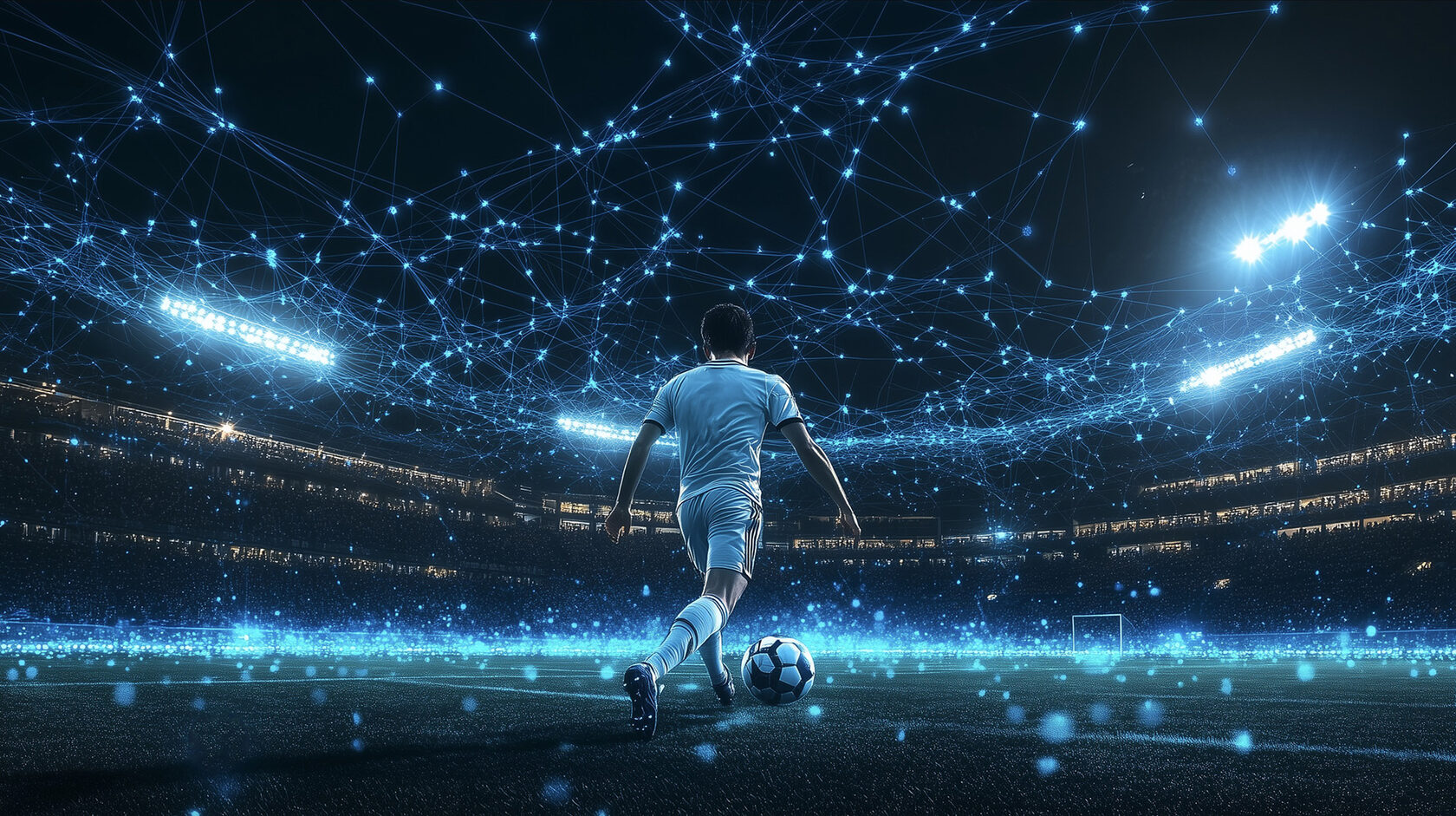
Tools and Technologies Used by CNR Analytics
To convert raw sports data into meaningful predictions, the CNR Analytics team relies on a powerful technical stack. They are simply programming languages like Python and R working hand-in-hand (or side-to-side). It is widely used for general data processing, machine learning model development, and automation scripts. Pandas, NumPy, scikit-learn are libraries that will get you started for data cleaning, and using data to create predictive models. When deep learning is required — for instance, when what’s needed is to analyze patterns in massive datasets or to model complex interactions — analysts use frameworks such as TensorFlow and PyTorch. Some team members also use R (mainly for statistical analysis and rich visualizations), with packages like dplyr, ggplot2, and caret assisting in rapid exploratory analysis and prototyping of models. That dual-language approach also provides the team some degree of flexibility, too: Python brings scale and integration with web services; R provides great statistical tools and plotting capabilities.
Behind the scenes, company analytics portal operate on a cloud system. The team works with cloud services (AWS, Google Cloud, etc.) to host databases and deploy machine learning models Historic match data, for instance, may be stored in an AWS S3 data lake or a relational database, so analysts can quickly query years of stats using SQL. Compute instances (like AWS EC2 or Google Cloud VMs) are able to spin-up on-demand power to run expensive computations needed to train a new predictive model on decades of sports data or to run thousands of simulations of an upcoming game (the Monte Carlo simulations) to compute win probabilities. Using the cloud set up also means the remote team can work asynchronously together: they share notebooks and code in a version controlled repository (e.g. GitHub or GitLab) and they all have secured access to the same centralised data resources.
The frameworks used for predictive modeling are state of the art. A variety of models are created by analysts based on the specific sport and question. Random forests, gradient boosted trees (XGBoost and similar libraries) are used to discover non-linear relationships between variables to determine, for example, how the combination of player fitness metrics, team form and match day weather conditions might impact on the outcome of a soccer match. For time-series forecasts (such as the in-game score progression in a cricket match, or point-by-point momentum shifts in a tennis match), they deploy models including ARIMA and LSTM neural networks. Classification models predict the discrete outcomes of a match (win, lose, draw) and regression models predict score-lines or spread points. The team often will build ensemble models that combine multiple approaches, which improves accuracy and robustness. They also use domain-specific frameworks: for instance, they propose a variant of the Elo rating system to dynamically rate teams and players in association football and basketball and specialized rate stats (such as cricket’s strike rate or basketball’s PER) that are built into models to forecast analytics.
All of these models require fuel which is data, and company has built several extensive historical data pipelines which constantly gathers and updates this fuel. They process data from different sources: official sports APIs, live score feeds, and even web scraping when necessary. Tools such as Apache Airflow or a custom script are used to manage the pipeline, pulling setup data at predetermined regular intervals (e.g. after the end of each day to update games played during the last day, or real-time updates when live events are executed). Once collected, there’s a need to clean and standardize the data — aligning player names, team identifiers, dates and statistics across various sources. The transformed clean data resides in one central place i.e. in a Data Warehouse. The team has also built a layer of analytical databases on top of the original raw data, where queries can be run to extract features (like a team's number of wins in a row, a boxer's reach advantage, a fighter's takedown average, etc.). This architecture gives analysts fast access to any specific data they need to put into their models, even if that data covers decades and multiple leagues.
In order to predict outcomes well, the team does not merely train models and leave them to their own devices. It is a perpetual process of validation and backtesting. Every predictive model is back-tested against historical seasons or prior tournaments to see how it would have done. This is where frameworks such as scikit-learn become so useful, with cross-validation methods that educate us on how well our model generalizes after being shown the data. The team also makes use of cloud-based notebooks and dashboards for monitoring model performance in real time. If they see that a model’s accuracy begins to drift (perhaps due to a change in a sport’s dynamics, or new data) the analysts retrain or recalibrate the model. They sometimes integrate new data sources — such as including player injury reports or live weather updates in the pre-game models for football and cricket — and must modify their data pipelines accordingly. The infrastructure is designed to be modular, so new sports or new analytical methods can be plugged in without disturbing the entire system.
In the end, there is also a suite of tools to get the predictions to the users. An internal system in the Сompany takes these outputs of the above analytics models (predicted probabilities, scores, or betting tips) and formats it for dissemination on the telegram and WhatsApp channels within them. To post updates at preset times, this system may utilize some Python automation or even a chatbot integrated with their respective APIs (Telegram and WhatsApp). The marketing and design team uses templates with data inputting methods: Nina (the designer) might fill out a template that then assembles a colourful summary of how predictions look for the day, and Mark (the web dev) makes that web portal change from predictions to predictions with a click. Everything else: All these technical tools and frameworks work in parallel, allowing a distributed team of experts to generate consistent analysis that is aligned through the shared model and hyper-performant.